Python for Data Science For Dummies, Mueller J.P., Massaron L., 2019.
The main purpose of Python for Data Science For Dummies, 2nd Edition is to take the scare factor out of data science by showing you that data science is not only really interesting but also quite doable using Python. You might assume that you need to be a computer science genius to perform the complex tasks normally associated with data science, but that’s far from the truth. Python comes with a host of useful libraries that do all the heavy lifting for you in the background. You don’t even realize how much is going on, and you don’t need to care. All you really need to know is that you want to perform specific tasks, and Python makes these tasks quite accessible.
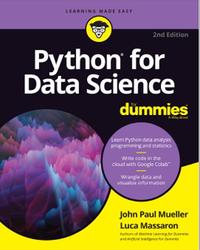
Ten Data Challenges You Should Take.
Data science is all about working with data. While working through this book, you have used a number of datasets, including the toy datasets that come with the Scikit-learn library. Of course, these datasets are all great for getting you started, but just as a runner wouldn’t stop after conquering the local fun run, so you need to start training for data science marathons by working with larger datasets.
This chapter introduces you to a number of challenging datasets that can help you become a world-class data scientist. By combining what you discover in this book with these new datasets, you can learn how to do amazing things. In fact, some people may view you as a bit of a magician as you pull seemingly impossible data patterns out of your hat. Each of the following datasets provides you with specific skills and helps you achieve different goals.
Contents.
Introduction.
Part 1: Getting Started with Data Science and Python.
Chapter 1: Discovering the Match between Data Science and Python.
Chapter 2: Introducing Python's Capabilities and Wonders.
Chapter з: Setting Up Python for Data Science.
Chapter 4: Working with Google Colab.
Part 2: Getting Your Hands Dirty with Data.
Chapter 5: Understanding the Tools.
Chapter 6: Working with Real Data.
Chapter 7: Conditioning Your Data.
Chapter 8: Shaping Data.
Chapter 9: Putting What You Know in Action.
Part 3: Visualizing Information.
Chapter 10: Getting a Crash Course in MatPlotLib.
Chapter 11: Visualizing the Data.
Part 4: Wrangling Data.
Chapter 12: Stretching Python's Capabilities.
Chapter 13: Exploring Data Analysis.
Chapter 14: Reducing Dimensionality.
Chapter 15: Clustering.
Chapter 16: Detecting Outliers in Data.
Part 5: Learning from Data.
Chapter 17: Exploring Four Simple and Effective Algorithms.
Chapter 18: Performing Cross-Validation, Selection, and Optimization.
Chapter 19: Increasing Complexity with Linear and Nonlinear Tricks.
Chapter 20: Understanding the Power of the Many.
Part 6: The Part of Tens.
Chapter 21: Ten Essential Data Resources.
Chapter 22: Ten Data Challenges You Should Take.
Index.
Купить .
Теги: учебник по программированию :: программирование :: Mueller :: Massaron
Смотрите также учебники, книги и учебные материалы:
- Python и наука о данных для чайников, Мюллер Д.П., Массарон Л., 2020
- Python для чайников, Мюллер Д.П., 2019
- Python для детей, самоучитель по программированию, Бриггс Д., 2017
- Python for Kids, A Playful Introduct to Programm, Briggs J.R., 2012
- JavaScript для детей, самоучитель по программированию, Морган Н., 2016
- JavaScript for Kids, Morgan N., 2014
- Introducing Python, Modern Computing in Simple Packages, Second edition, Lubanovic B., 2020
- Introducing Python, Modern Computing in Simple Packages, Lubanovic B., 2015